Your Organization Isn’t Designed to Work with GenAI
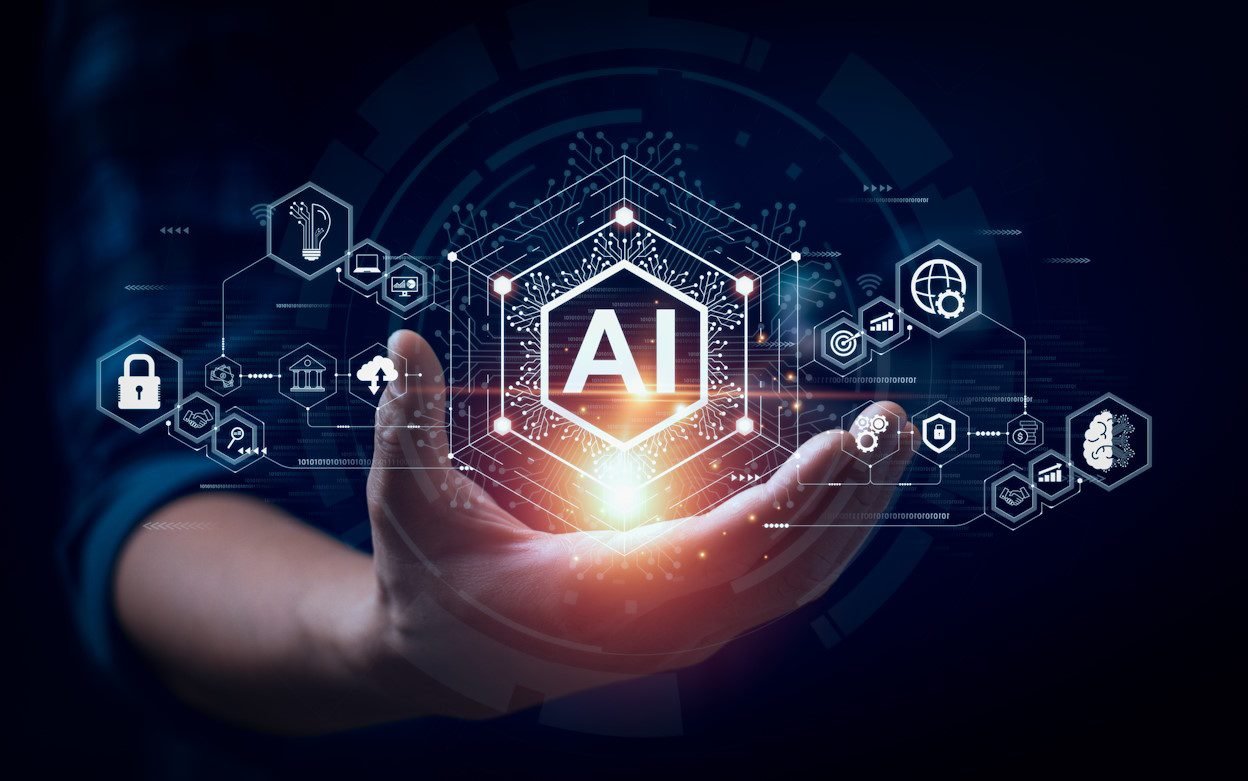
Organizations are plowing millions of dollars into generative AI as they race to apply it in innovative ways ahead of the competition. Yet many are hitting roadblocks, not due to the known shortcomings of the technology, which is still in its infancy, but because of a fundamental flaw in their approach: They think of GenAI as a traditional form of automation rather than as an assistive agent that gets smarter — and makes humans smarter — over time.
The introduction of the internet, mobile computing, and cloud platforms showed us that extracting full value from groundbreaking technologies lies not in merely integrating them into existing business processes, but in completely reimagining those processes. While GenAI may prove even more transformative than these innovations, it similarly demands redesigning the way work gets done to maximize its potential.
The problem companies are facing, however, is that traditional methods of process redesign may not be entirely up to the task because GenAI doesn’t function like a traditional technology. Users “talk” to it, much as they would to a human colleague, and it works with the user in an iterative fashion. It also can continuously improve as it learns user needs and behaviors (and vice versa).
To effectively integrate GenAI, we propose a new paradigm: Designing for Dialogue. Unlike traditional, technology-driven process redesign principles that focus on taking capabilities “out” of the human and putting them “into” the machine, Designing for Dialogue is rooted in the idea that technology and humans can share responsibilities dynamically. Each takes the lead at different points throughout a process based on context and competence. It essentially treats GenAI more like a coworker than a static technology. Make no mistake: We aren’t suggesting that GenAI is human or sentient — only that it should be treated differently than other technologies because it behaves more like a colleague than previous software.
By designing for dialogue, organizations can create a symbiotic relationship between humans and GenAI. The approach also provides the flexibility for the process to become more efficient over time, almost organically. And every more-efficient process that emerges can be captured and examined for potential future automation, putting organizations on a path to continuous improvements and efficiency gains.
Why old reengineering methods won’t work
Back in 1990 the Harvard Business Review published a breakthrough article by the late Michael Hammer: “Reengineering Work: Don’t Automate, Obliterate.” It laid out the fundamentals of business process reengineering (BPR), which emphasizes the rethinking and radical redesign of business processes to achieve significant improvements in efficiency, quality, service, and speed. The approach calls for dissecting and understanding the entirety of a business’s workflows and reconstructing them from the ground up for optimal performance.
When performed to take advantage of a new technology, BPR is typically led jointly by IT and the business. A working team assigns tasks previously performed by humans to the new technology based on the technology’s fixed capabilities. The team redefines the workflow around these static automations and, likely with significant change management, employees adopt the redesigned process. Ideally, the implementation team then monitors the new process, tweaking it periodically for continuous improvement, but not undertaking frequent, wholesale redesigns. The BPR method has worked for businesses for decades and is still predominantly employed in digital transformations today.
While BPR’s goals and task-based philosophy can certainly apply to GenAI, its top-down approach aimed at both rigidly assigning tasks to either humans or technology and creating a fixed process fails to capitalize on GenAI’s flexible, iterative nature. Unlike previous technologies, GenAI facilitates a dynamic interaction and bidirectional feedback loop between human and machine. In other words, the AI and human perform a task together, learn from and improve each other, and continuously optimize a process at the user level in near-real-time. When integrating GenAI, the implementation team, therefore, becomes more of a facilitation team.
A better way: Designing for Dialogue
When we watch a high-performing human team in action, we see a dynamic collaboration: A project manager delegates tasks, but team leadership fluidly changes hands based on who can best address the challenge of the moment. Spontaneous brainstorming sessions lead to innovative solutions. Teammates seek counsel from each other for their unique areas of expertise, building up each other’s’ knowledge — and team performance — over time. The Design for Dialogue framework mirrors these human dynamics to create an effective, collaborative, and adaptable human–AI workflow.
At the heart of the framework are three primary components: task analysis, interaction protocols, and feedback loops.
A rigorous task analysis, akin to a project manager’s assessment of who is best suited for a particular role within a team, involves breaking down a process into its component tasks and evaluating the complexity, risks, and capabilities required for each. This ensures that each task is assigned to the right leader — AI or human.
Interaction protocols — the equivalent of team ground rules — outline how AI and humans communicate and collaborate rather than establish a fixed process. One example would be when AI encounters an anomaly or a strategic decision point, it signals for human judgment, much like a team member would seek advice when faced with a hurdle. Another could be that proactive suggestions from AI serve as starting points for discussion, inviting human input to refine the course of action, rather than a steadfast command.
Finally, just as teams debrief and adjust their approach in daily standup calls, or at least at each stage of a project, organizations will want to put mechanisms in place to continuously assess and fine-tune AI–human collaboration based on feedback. These could include error reporting, success metrics, user satisfaction surveys, and AI decision-making explanations.
A good example is the customer service model employed by Jerry, a company valued at $450 million with over five million customers that serves as a one stop-shop for car owners to get insurance and financing. Jerry receives over 200,000 messages a month from customers. With such high volume, the company struggled to respond to customer queries within 24 hours, let alone minutes or seconds. By installing their GenAI solution in May 2023, they moved from having humans in the lead in the entirety of the customer service process and answering only 54% of customer inquiries within 24 hours or less to having AI in the lead 100% of the time and answering over 96% of inquiries within 30 seconds by June 2023. They project $4 million in annual savings from this transformation.
To achieve it, they broke down the customer service agent’s role into knowledge domains — for example, quoting, binding and payments for insurance — and tasks, such as fielding an initial inquiry, assessing the nature of the inquiry, pulling up the correct source of information to find an answer, finding the specific user’s information, and so on. They discovered that while AI can take the lead in performing much of this work, there are points in the AI–customer interaction when matters need to be escalated to the agent, who then takes the lead. Interaction protocols determine when that should occur; for example, if AI detects negative sentiment from the customer, the AI can’t parse what the customer needs from their inputs into the chat, or the AI determines it can’t confidently provide an answer.
Feedback loops are critical and used to refine the customer service process on a near-daily basis. Agents have been trained to identify issues and enter tickets into a tracking system, customers can click a thumbs up or thumbs down on an interaction, and instances of escalation are automatically flagged by the system for investigation. Engineers review failures daily and adjust the system to correct them.
In addition to improving customer service and satisfaction, they’ve increased job satisfaction among human agents because AI conducts the laborious hunt for information regarding escalated issues and presents the agent with context and a clear course for action. Agents can also query the system directly to easily find additional information. And thanks to Jerry’s continued growth, they haven’t decreased the number of agents. Plus, the rich data Jerry collects from customer interactions translate into ways to improve other aspects of their business, such as by offering additional services aligned to customer needs and more targeted marketing and upselling.
We’ve also used the Design for Dialogue approach at our research firm for the process of crafting reports. We broke down the process into its individual tasks and determined whether AI or a human takes the lead for each. Humans take the lead for tasks requiring human judgment, such as determining research focus and verifying information. We’ve established interaction protocols, such as requiring analysts to verify sources for any information provided by AI. And we’ve gathered user feedback to refine the process. For example, while we initially put AI in the lead for data visualization, we learned we could create charts more efficiently with humans providing more initial guidance.
How to get started
Redesigning your organization’s processes to incorporate GenAI can be transformative — with the right approach. The steps outlined here can help ensure success.
Identify high WINS work processes that create the most value for your organization.
Begin with a thorough assessment of existing workflows, identifying areas where AI could have the most significant impact. Processes that involve a high degree of work with words, images, numbers, and sounds — what we call WINS work are ripe for providing humans with GenAI leverage. They can most often be found in customer service, sales and marketing, software engineering, and research and development.
Perform task analysis.
Understand the sequence of actions, decisions, and interactions that define a business process. For each identified task, develop a profile that outlines the decision points, required expertise, potential risks, and contextual factors that will influence the AI’s or humans’ ability to lead. Consider where human expertise is irreplaceable, particularly in areas requiring emotional intelligence, complex decision-making, and nuanced judgments.
Design interaction protocols and feedback mechanisms.
Define how AI systems should engage with human operators and vice versa, including establishing clear guidelines for how and when AI should seek human input and vice versa. Develop feedback mechanisms, both automated and human led.
Train the team.
Conduct comprehensive training sessions to familiarize employees with the new AI tools and protocols. Focus on building comfort and trust in AI’s capabilities and teach how to provide constructive feedback to and collaborate with AI systems.
Evaluate, adjust, and scale.
Roll out the AI integration with continuous monitoring to capture performance data and user feedback and refine the process. Continuously update the task profiles and interaction protocols to improve collaboration between AI and human employees while also looking for process steps that can be completely automated based on the interaction data captured. Once the initial integration is successful and the processes have been refined, consider scaling to other areas of the organization, adapting the framework to different contexts and needs. Jerry, for example, is now working on applying their new model to phone interactions.
Conclusion
Three to five years from now, organizations will have conversational interfaces for all types of processes, products, and services. Those that develop deep expertise in designing for dialogue will not only have a competitive advantage but will also gather all types of new interaction data that they can use to improve their existing products and innovate new ones.
In essence, the Design for Dialogue framework is about more than optimizing workflows; it’s about reshaping the very fabric of collaboration in the AI-powered age. Yes, GenAI can automate tasks and augment human capabilities. But reimagining processes in a way that utilizes it as an active, learning, and adaptable partner forges the path to new levels of innovation and efficiency.
Source: GWFM Research & Study